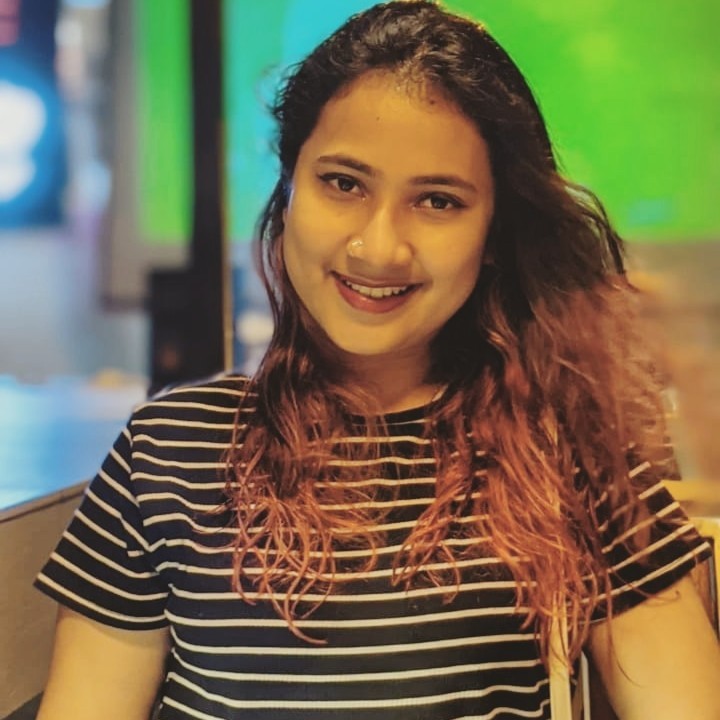
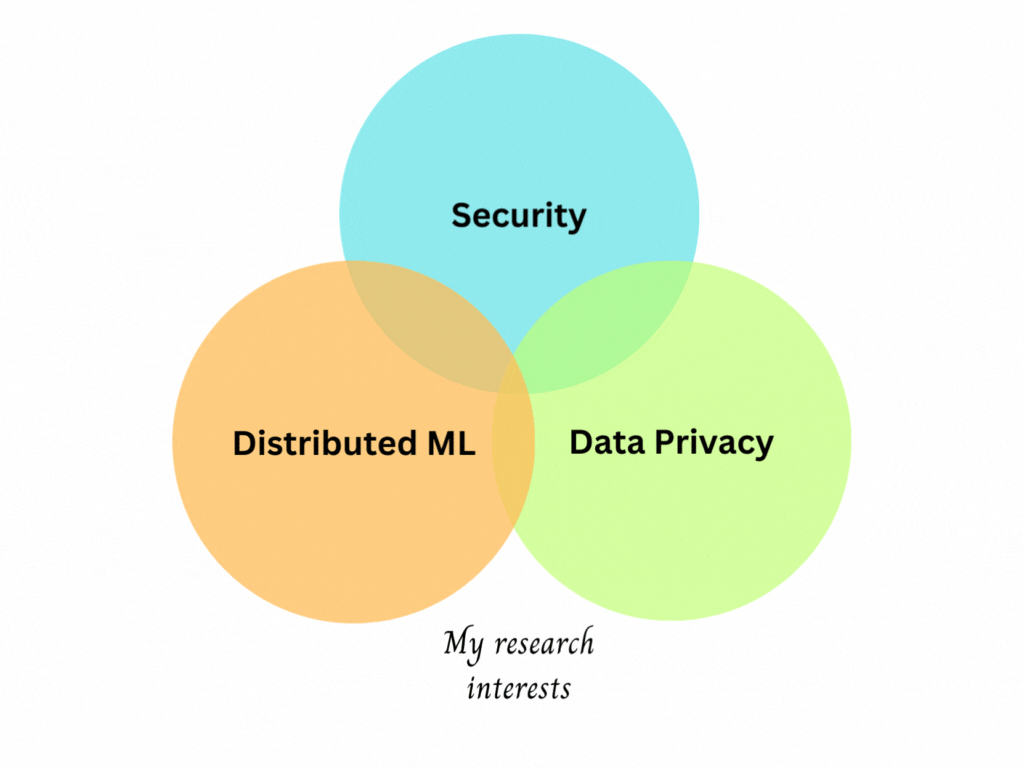
Mushtari Sadia
About me
I'm Sadia (she/her), a fresh graduate from the department of CSE, BUET. I am currently working as a lecturer in the department of CSE, BRAC University, and previously worked as an adjunct lecturer at the department of CSE, BUET. My research interests lie in the fields of Computer Security, Privacy, as well as Distributed Machine Learning.
My current career goal is to pursue a PhD program in my field of interest. If all goes well, I will be joining the CSE, PhD program at the University of Michigan-Ann Arbor in Fall 2024!
Currently I’m working with Dr. Praneeth Vepakomma (MIT) on implementing the concept of layer parallelization of the training process of deep learning models, something that could highly impact the field of Federated Learning. I’ve also previously worked on independent research projects in this area, particularly one where I implemented a secure-sum gradient descent protocol for Federated Learning using Docker.
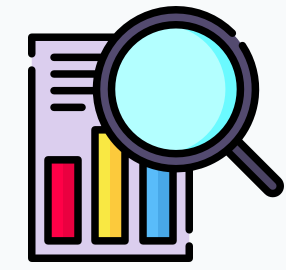
Apart from my research, I am also fortunate enough to contribute to the academic community through teaching. I’m currently the instructor of undergraduate-level courses on Data Structures and Algorithms, Embedded Systems and Interfacing etc.
As an undergrad student at BUET, I've participated and won multiple prestigious competitions in the fields of Machine Learning, Deep Learning, Natural Language Processing and Software Development such as Herwill Datathon 2022, Microsoft Virtual Hackathon 2022 and the UNDP WDI Hackathon 2021 as part of a team. I’m also passionate about the advancement of women in STEM in my country. I was the president of Bangladeshi Women in Computer Science and Engineering (BWCSE), where we initiated many projects to guide undergrad female students in my university to build their career in CSE.
I also love travelling, dancing and binge-watching sitcoms.
Work Experience
-
Lecturer (Full-time)
Department of Computer Science and Engineering,
BRAC University
June 2023 — PresentCourse Instructor:
(Summer 2023, Fall 2023, Spring 2024) CSE 220: Data Structures
(Summer 2023, Fall 2023, Spring 2024, Summer 2024) CSE 221: Algorithms
(Summer 2023, Fall 2023, Summer 2024) CSE 220: Data Structures Sessional
(Summer 2023, Spring 2024, Summer 2024) CSE 110: Programming Language I Sessional
-
Adjunct Lecturer
Department of Computer Science and Engineering,
Bangladesh University of Engineering and Technology
November 2023 — March 2024Course Instructor:
CSE391: Embedded Systems and Interfacing
CSE392: Embedded Systems and Interfacing Sessional
CSE102: Structured Programming Language Sessional
CSE412: Simulation and Modeling Sessional
-
Research Fellow
August 2023 — February 2024Worked with Dr. Praneeth Vepakomma (MIT) on a distributed machine learning project, implementing the concept of layer parallelization of the training process of deep learning models.
Research Experience
-
Effectiveness of Transformer-based Language Models in Detecting Advanced Persistent Threats from System Provenance Graphs (2022 - Current)
Computer Security, Natural Language ProcessingUndergraduate thesis project under Dr. Anindya Iqbal and Dr. Shahrear Iqbal(Research Officer, National Research Council (NRC) Canada). In this work, I co-implemented a framework which includes a robust process of creating a provenance graph from raw log data from the DARPA OPTC and DARPA TC E3 datasets, subsequently generating event sequences from that graph and finally transforming the data into a suitable format for transformer-based language models such as BERT, RoBERTa, GPT. My personal contributions were building the postgres database from the raw log datasets, designing SQL queries to generate the provenance graph, co-writing the code for preprocessing the graph data to extract traces with relevant attributes, and finally building the experiments with various pre-trained LLMs. We were able to achieve state of the art performance from our framework in detection of APT attacks. Currently, this work is under review for publication.
Read the preprint: [arXiv] -
Advancing Parallelization in Deep Learning Training: A Novel HSIC-Based Approach and Its Comparative Performance Against Traditional Federated Models (2023 - Current)
Federated Learning, Privacy, OptimizationWorking with Dr. Praneeth Vepakomma (MIT Camera Culture Group) as part of the Fatima Fellowship Research Program, on a distributed machine learning project. We developed a method for parallelizing the forward propagation in neural network training, utlizing the HSIC objective function to eliminate the need for backpropagation, all while maintaining the same level of accuracy. We also discovered that, in some instances, employing slightly outdated local updates can signifcantly reduce communicaton costs without compromising accuracy. We are presently in the phase of manuscript review of this work, as we prepare it for publication.
-
Development of Flood Forecasting System for Bangladesh-India Using Different Machine Learning Techniques (2020)
Machine LearningIn this study, I preprocessed datasets of weather parameters and employed the use of five different machine learning algorithms- exponent back propagation neural network (EBPNN), multilayer perceptron (MLP), support vector regression (SVR), DT Regression (DTR), and extreme gradient boosting (XGBoost), which were used to develop total 180 independent models based on a different combination of time lags for input data and lead time in forecast. Models were developed for Someshwari-Kangsa sub-watershed of Bangladesh’s North Central hydrological region with 5772 km2 drainage area.
EGU General Assembly 2021: [Poster Presentation] -
Dengue Forecasting System (2020-2022)
Machine LearningWorked with Dr. ABM Alim Al Islam, Ramisa Alam, Mashiat Mustaq and Tahiea Taz. In this project, we built a time series forecasting model-based dengue forecast system that predicts the number of dengue cases in any given region based on the recent cases in that region and the state of different weather parameters. My contributions in the project was preprocessing the datasets as well as developing the models using MS Azure Services.
Presented the idea and won the 1st Runner Up position in Microsoft Virtual Hackathon 2022.
Education
-
B.Sc. in Computer Science and Engineering
Bangladesh University of Engineering and Technology
April 2018 - May 2023CGPA: 3.84/4.00
Notable Courses:
CSE471- Machine Learning CSE405- Computer Security CSE453- High Performance Database Systems CSE309- Compiler Design CSE321- Computer Networks CSE313- Operating Systems CSE463- Introduction to Bioinformatics CSE409- Computer Graphics CSE411- Simulation and Modeling MATH247- Linear Algebra MATH245- Statistics and Probability CSE305- Computer Architecture -
Higher Secondary School Certificate (HSC)
Engineering University School and College
2017GPA: 5.00/5.00
- Board General Scholarship
-
Secondary School Certificate (SSC)
Engineering University School and College
2017GPA: 5.00/5.00
Technical Skills
-
Programming Languages
C/C++, x86 Assembly, Bison/Flex, Python, Java, Javascript, Bash, MySQL
-
Frameworks
Docker, PyTorch, NS3, xv6, Django REST, ReactJS, Git, Oracle DBMS, LaTeX, Wireshark
-
Libraries
Sklearn, Pandas, Matplotlib, Seaborn
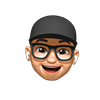